In a recent review published in the journal Fishes, the authors explored the applications of artificial intelligence (AI)-based tools in studying fishing fleet behavior, recognizing the crucial role of monitoring fishing vessels (FV) in fisheries management and ecological equilibrium.
With the proliferation of real-time data from vessel-tracking systems, AI algorithms have become essential for efficiently analyzing extensive datasets. The present review begins by introducing diverse data sources for studying FV behavior, followed by a comparative analysis of their strengths and limitations. It also explores studies on the physical, ecological, and social mechanisms influencing FV behavior, contributing to a comprehensive understanding.
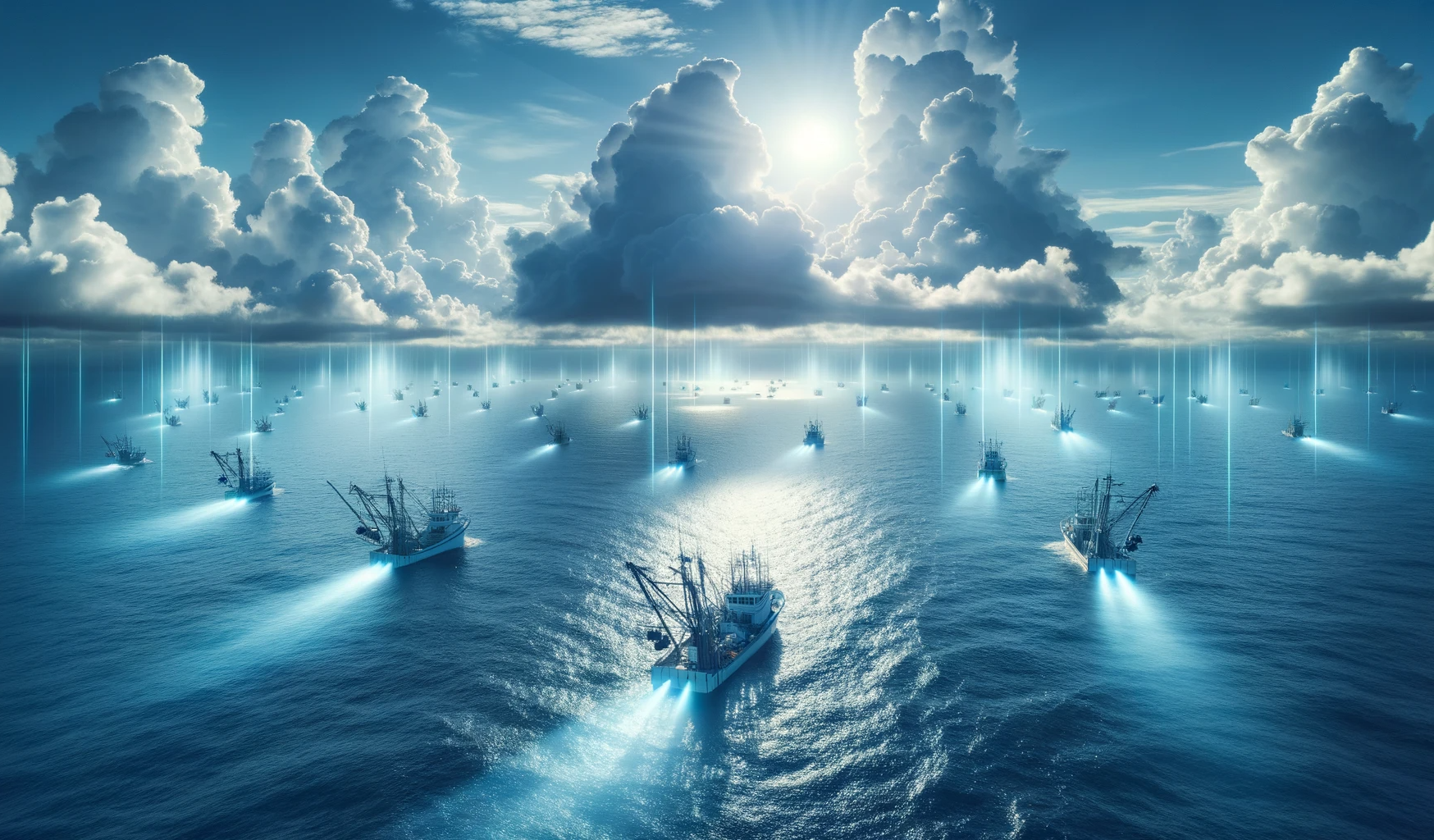
Study: AI-Based Tools for Studying Fishing Fleet Behavior. Image credit: Created with the assistance of DALL·E 3
Sources for FV Behavior Research
The following are the data sources used in FV research:
Fisheries Logbook: The fishery logbook serves as a fundamental carrier document detailing navigation conditions and fishing activities. Typically maintained by the captain or designated personnel, it proves most effective when combined with other monitoring methods. Its cost-effectiveness, especially in electronic form, enhances convenience. However, its reliability hinges on the recorder's diligence and the fishing ground's context, leading to potential data incompleteness and inaccuracy.
Fisheries Observers: Maritime observers, authorized to monitor fishing activities, are classified as scientific or law enforcement observers. The former collects catch, biological, and production data for resource assessment, while the latter ensures compliance with fishing regulations. Despite being one of the most effective means for accurate data collection, the maritime observer program is logistically complex and costly.
Vessel Monitoring System (VMS): VMS, established by international fishery entities or nations, incorporates a transmitter, satellite transmission system, and ground receiver. Offering real-time FV information aids in monitoring and law enforcement. Its trajectory data analysis contributes to comprehensive fisheries management.
Automatic Identification System (AIS): AIS, a navigational assistance system, facilitates ship identification, positioning, and collision avoidance. It generates extensive real-time FV information with characteristics of big data. Widely applied in marine governance, safety, fishing ground research, and behavior identification, AIS marks the digital era in marine operations and management.
AI Techniques Used in Monitoring FV Behavior
Data Standardization: Utilizing big data technology, preprocessing VMS or AIS data addresses errors. Invalid and abnormal data are removed, and normalization methods ensure standardized, reliable datasets.
Feature Extraction: Machine learning-based feature extraction involves clustering (e.g., hierarchical, K-means), dimensionality reduction, and feature importance analysis (e.g., logistic regression, random forest) to identify crucial FV behavior characteristics.
Dynamic Monitoring of FV: Advanced AI, particularly deep learning, enhances real-time detection and analysis of FV activities. Neural networks and algorithms improve efficiency and accuracy, crucial for safety and combating illegal practices.
Other than the above, AI-driven methods applied to VMS and AIS data enhance accuracy in identifying FV operation types. Feature fusion algorithms and Bayesian models contribute to robust monitoring, aiding in management practices and informing sustainable fishery planning.
Uses of AI in Studying FV Behavior
Identification of Vessel Types
Fishery management's effectiveness hinges on the ability to classify various FV types due to their distinct impacts on marine ecosystems. Machine learning approaches involve logistic regression, light gradient boosting, and spatial-temporal analysis, enhancing the accuracy of classifying FV types. Deep learning methods, such as recurrent neural networks and ensemble learning, have been particularly successful in this classification task, providing valuable insights for decision-making in fishery resource development.
Speculation of Fishery Resource Dynamics
Dynamic projections of fishery resources are critical for sustaining marine ecosystems and ensuring optimal resource utilization. Fishing forecasts, facilitated by AI, involve the development of prediction models using data on marine environments, meteorology, and fish behavior. AI-based algorithms, such as elevated regression tree models and hybrid prediction architectures, have demonstrated superior accuracy in forecasting fishing conditions. This enhances the efficiency of fishery production and contributes to sustainable fisheries management.
Fishing Density Forecast
The analysis of fishing activity intensity, or fishing density, provides crucial insights into the temporal and spatial distribution of fishery resources. Utilizing AIS and VMS data, AI algorithms, including Gaussian mixture models, deep residual networks, and convolutional networks, offer sophisticated means to study fishing intensity. These methods contribute to the dynamic guidance of fishery management, offering valuable information for the restoration and sustainable management of fishery resources.
Conclusion
To summarize, this review examines the integration of AI in analyzing FV behavior, emphasizing its success in identifying vessel types, detecting abnormal behaviors, and predicting fishery resources. The paper highlights AI's crucial role in enhancing safety, improving port management efficiency, and supporting marine ecological protection. Significantly, the qualitative leap achieved through AI's marriage with FV analysis is acknowledged, with a call for global efforts in trajectory data acquisition and standardized processing. The paper envisions a future where refined AI contributes to a smart fishery operation system, promoting transparent and intelligent fisheries and making a valuable contribution to sustainable marine resource management.
Journal reference:
- Cheng, X., Zhang, F., Chen, X., & Wang, J. (2023). Application of Artificial Intelligence in the Study of Fishing Vessel Behavior. Fishes, 8(10), 516. https://doi.org/10.3390/fishes8100516, https://www.mdpi.com/2410-3888/8/10/516
Article Revisions
- Oct 24 2023 - Minor corrections to punctuation and main image changed