In an article recently submitted to the arxiv* server, researchers introduced AudioSeal, an innovative audio watermarking technique tailored for localized detection of artificial intelligence (AI)-generated speech. They employed a generator/detector architecture, incorporating a novel perceptual loss for enhanced imperceptibility. AudioSeal demonstrated state-of-the-art performance in robustness against audio manipulations and speed, with a single-pass detector significantly outpacing existing models, making it suitable for large-scale and real-time applications.
Study: AudioSeal: Unveiling a Sonic Sentry for AI-Generated Speech Detection. Image credit: Tapati Rinchumrus/Shutterstock

*Important notice: arXiv publishes preliminary scientific reports that are not peer-reviewed and, therefore, should not be regarded as definitive, used to guide development decisions, or treated as established information in the field of artificial intelligence research.
Background
Generative speech models have reached a point where they can create voices indistinguishable from real ones, raising concerns about misinformation and scams. Current detection methods, relying on binary classifiers to differentiate natural and synthesized audios, face challenges as generative models improve. Watermarking, embedding an invisible signal, emerges as an alternative for traceability and content identification.
Traditional detection methods, though accurate initially, struggle with advancing generative models. Watermarking methods, while promising, lack adaptation for effective detection and often lack localization, hindering the identification of small AI-generated segments within longer audio clips. This paper introduced AudioSeal, a novel audio watermarking technique tailored for localized AI-generated speech detection at the sample level.
By training a generator and a detector simultaneously, AudioSeal overcame the limitations of existing methods. The generator predicts imperceptible watermarks, and the detector precisely identifies synthesized speech in longer clips. A unique perceptual loss enhances imperceptibility, and AudioSeal excels in speed and robust detection, introducing multi-bit watermarking for model attribution without compromising detection. The contributions include a pioneering training approach, achieving state-of-the-art detection and localization results, and substantial computation speed improvements, making it ideal for real-time applications.
Methods
The proposed method, AudioSeal, employed a joint training approach for a generator and a detector. The generator added an imperceptible watermark to the input audio while the detector outputted local detection logits. Training involved minimizing perceptual distortion and maximizing watermark detection concurrently. Augmentations enhanced robustness to signal modifications and localization. At inference, the detector precisely localized watermarked segments, enabling the detection of AI-generated content.
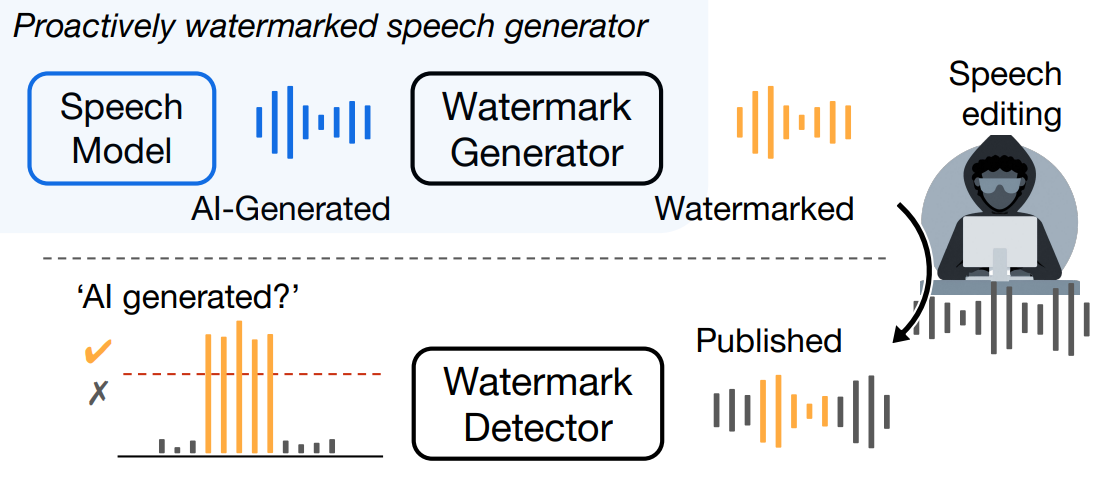
Proactive detection of AI-generated speech. We embed an imperceptible watermark in the audio, which can be used to detect if a speech is AI-generated and identify the model that generated it. It can also precisely pinpoint AI-generated segments in a longer audio with a sample level resolution (1/16k seconds).
- Training Pipeline: The training process involved watermark generation, sample-level localization augmentation, robustness augmentation, and detector processing. The architecture was based on EnCodec, emphasizing imperceptibility and accurate localization.
- Losses: Various perceptual losses ensured imperceptibility, including multi-scale Mel spectrogram loss and adversarial losses. A novel time-frequency (TF) loudness loss, TF-Loudness, enhanced watermark imperceptibility based on auditory masking. Additionally, a masked sample-level detection loss ensured precise localization.
- Multi-bit Watermarking: The method extended to multi-bit watermarking for model attribution. A message processing layer in the generator and linear layers in the detector support embedded and extracted binary messages, attributing audio to specific model versions.
- Training Details: The models were trained on a VoxPopuli dataset subset with a sampling rate of 16 kHz. Training involved 600 thousand steps with Adam optimizer, a learning rate of 0.0001, and batch size of 32. Augmentations included drop and robustness augmentations. The perceptual losses were balanced, and the localization and watermarking losses were appropriately weighted.
Audio/Speech Quality
AudioSeal performed detection, localization, and attribution. Evaluation metrics included perceptual measures and a subjective MUSHRA test, demonstrating AudioSeal's focus on perceptual quality rather than scale invariant signal-to-noise ratio (SI-SNR) optimization. AudioSeal achieved comparable or better perceptual quality than traditional watermarking methods like WavMark, showcasing its effectiveness in imperceptible watermarking for speech signals.
Experiments and Evaluation
The detection performance of passive classifiers, watermarking methods, and AudioSeal was assessed, with true positive rate (TPR) and false positive rate (FPR) as key metrics for watermark detection. A comparison with a passive classifier, Voicebox, showed AudioSeal's superior performance, achieving perfect classification across various scenarios.
- Comparison with Passive Classifier: AudioSeal's proactive detection outperformed Voicebox in distinguishing between original and AI-generated audio across different masking levels. Additionally, when compared on the task of distinguishing between Voicebox and re-synthesized audio, AudioSeal maintained perfect detection, emphasizing its robustness against classifier-specific artifacts.
- Comparison with Watermarking Methods: Evaluation against robustness and audio editing attacks, including time modification, filtering, audio effects, noise, and compression, demonstrated AudioSeal's superior robustness compared to WavMark. The average area under curve (AUC) for AudioSeal was 0.97, outperforming WavMark's 0.84, indicating its effectiveness in diverse scenarios.
- Localization and Attribution: AudioSeal excelled in localization accuracy, achieving a high Intersection over Union (IoU) even with minimal watermarked speech duration. In attribution tasks, AudioSeal's accuracy surpassed WavMark, demonstrating its ability to identify the model version that generated the audio effectively.
- Efficiency Analysis: In terms of efficiency, AudioSeal significantly outperformed WavMark, being 14 times faster in generation and two orders of magnitude faster in detection. This efficiency and accurate detection and attribution positioned AudioSeal as a viable solution for real-time and large-scale applications.
Conclusion
In conclusion, AudioSeal represented a novel approach to audio watermarking, focusing on localized detection rather than traditional data hiding. By employing a generator/detector architecture, it achieved state-of-the-art robustness against audio editing techniques, precise localization, and significantly faster runtime.
The method was ready for practical deployment in voice synthesis applications programming interfaces (APIs), addressing content provenance concerns. However, ethical considerations arose regarding potential misuse, emphasizing the need for robust security measures and legal frameworks to govern the technology's application despite its essential role in detecting AI-generated content for transparency and traceability.

*Important notice: arXiv publishes preliminary scientific reports that are not peer-reviewed and, therefore, should not be regarded as definitive, used to guide development decisions, or treated as established information in the field of artificial intelligence research.
Journal reference:
- Preliminary scientific report.
Roman, R. S., Fernandez, P., Défossez, A., Furon, T., Tran, T., & Elsahar, H. (2024, January 30). Proactive Detection of Voice Cloning with Localized Watermarking. ArXiv.org. https://arxiv.org/abs/2401.17264
Article Revisions
- Feb 5 2024 - Inclusion of a figure from the research paper