Despite advances in AI, state-of-the-art vision-language models falter in abstract reasoning, highlighting new challenges in the quest for human-like cognition.
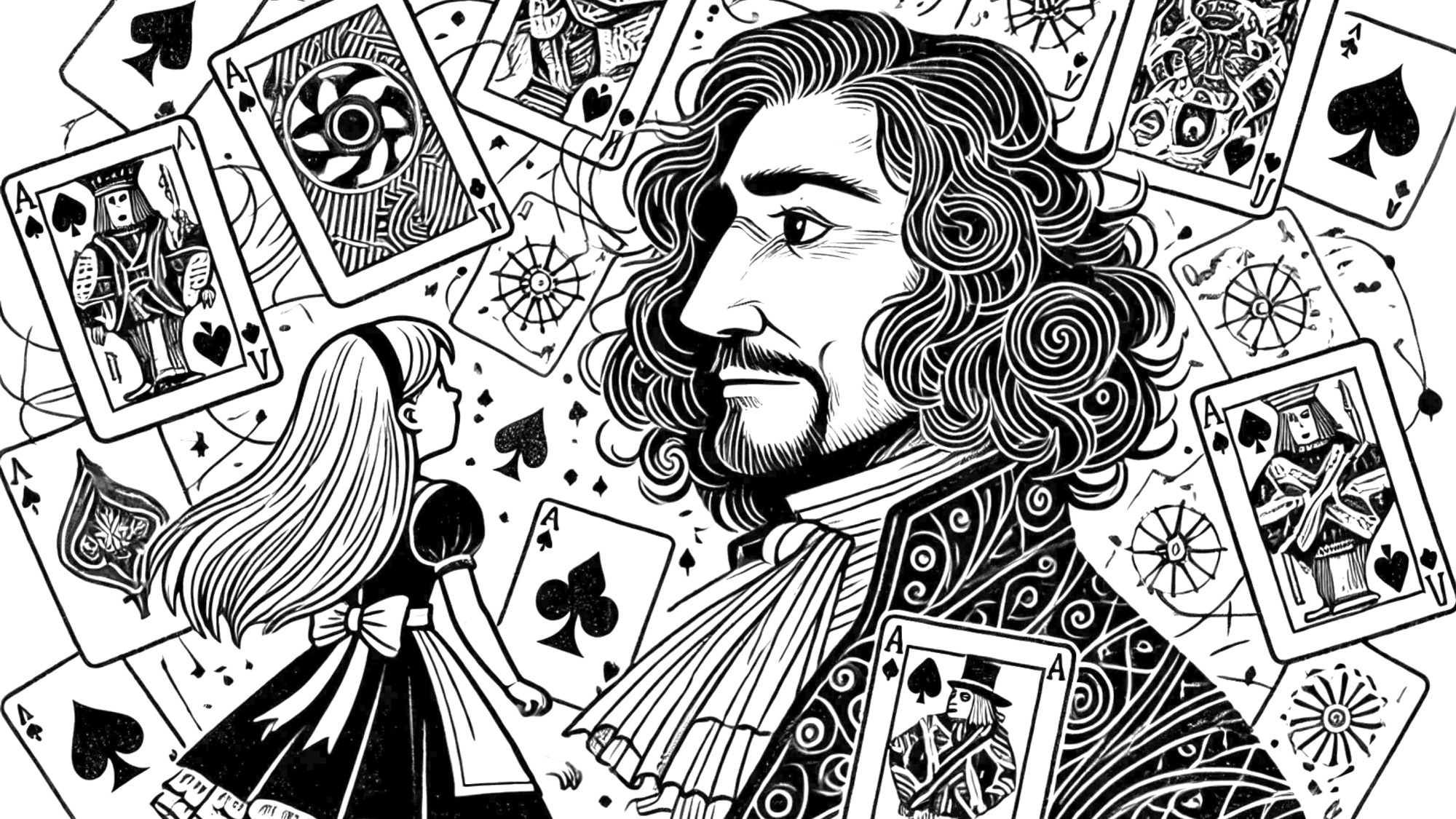
The wonderland of Bongard problems. The challenging puzzles of Bongard circle around simple black and- white diagrams that picture geometric shapes, patterns, or lines arranged in various configurations. While the visualization is rather simple, the underlying concepts can be abstract and complex. Study: Bongard in Wonderland: Visual Puzzles that Still Make AI Go Mad? Image Credit: Shutterstock AI

*Important notice: arXiv publishes preliminary scientific reports that are not peer-reviewed and, therefore, should not be regarded as definitive, used to guide development decisions, or treated as established information in the field of artificial intelligence research.
An article recently posted on the arXiv preprint* server explored the capabilities of Vision-Language Models (VLMs) in solving Bongard problems. These visual puzzles require advanced pattern recognition and abstract reasoning, serving as a highly challenging benchmark for evaluating AI progress in visual reasoning.
The researchers aimed to assess how well these models can replicate human-like visual reasoning and abstract thinking, especially regarding pattern recognition and conceptual understanding, while also identifying significant limitations.
Advancement in Artificial Intelligence (AI)
VLMs represent a significant advancement in AI by integrating visual and textual information to facilitate sophisticated reasoning tasks. These models, including OpenAI's GPT-4o (generative pre-trained transformer version 4 optimized for multimodal input), are designed to process both images and text, allowing them to generate descriptive content that seems to reflect human-like understanding.
However, recent studies have revealed notable shortcomings in VLMs, particularly in their ability to perform complex reasoning tasks that require an understanding of spatial and abstract visual concepts. This research focuses specifically on Bongard Problems, introduced by Mikhail Bongard in 1967. These problems consist of pairs of images that share a common rule, requiring models to identify their underlying conceptual differences. Such tasks involving complex relational reasoning serve as a unique and challenging benchmark for evaluating the visual reasoning capabilities of AI systems.
Exploring Various VLMs for Bongard Problems
In this paper, the authors investigated the performance of various state-of-the-art VLMs in solving Bongard Problems, aiming to clarify their strengths and critical limitations in visual reasoning. They systematically divided their analysis into two main settings: an open-ended approach and a multiple-choice format.
In the open-ended setting, models analyzed twelve diagrams and deduced the specific rules governing each side. The study provided detailed instructions, guiding the models through a step-by-step evaluation process, which included a methodical analysis of the diagrams and a structured response format.
In the multiple-choice setting, VLMs received predefined rule pairs and were tasked with selecting the correct one based on the features presented in the diagrams. This approach aimed to simplify problem-solving while assessing the models' ability to effectively recognize and apply learned concepts.
The researchers compared the performance of several VLMs, including OpenAI’s GPT-4o, Anthropic’s Claude, Gemini 1.5, and Large Language and Visual Assistance (LLaVA) models, against human performance to establish a baseline for evaluating the models' reasoning capabilities. The goal was to quantify how well VLMs could solve Bongard Problems compared to human participants and to identify specific areas where these models struggle, particularly in recognizing basic visual concepts and applying complex, abstract reasoning.
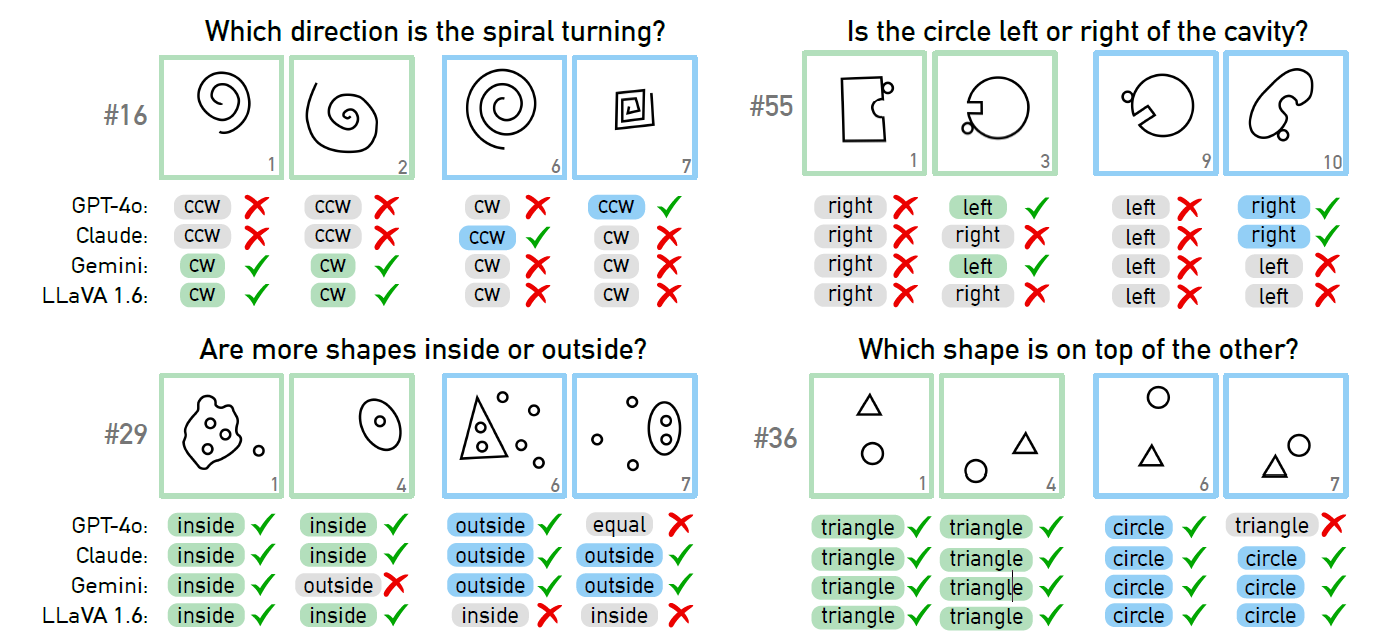
VLMs fail to identify simple visual concepts. VLMs challenged with identifying visual concepts in BPs. Although the VLM is able to recognize some of the concepts when specifically asked for (bottom), on the others, it continues to falter (top). Abbreviations used for clockwise (cw) and counter-clockwise (ccw).
Key Findings and Insights
The outcomes showed a significant gap between human and machine performance in solving Bongard Problems. The best-performing model, GPT-4o, solved only 21 of 100 problems, indicating a substantial deficiency in its reasoning capabilities. In contrast, human participants demonstrated an average accuracy of 84.41%, highlighting the challenges VLMs face in tasks requiring a nuanced understanding of visual patterns.
The study further highlighted that while VLMs occasionally succeeded in identifying discriminative concepts, they often struggled to understand and reason about relational visual elements. For example, even simple concepts, such as the direction of shapes like spirals, presented considerable challenges for the models. The results indicated that VLMs struggled with direct pattern recognition and the conceptual abstraction needed to formulate rules that differentiate between visual groups.
Additionally, the authors found that models performed better in the multiple-choice format, especially when the number of options was reduced. This suggests that while VLMs may struggle with open-ended reasoning tasks, they can achieve higher accuracy when given specific choices, likely due to a reliance on elimination strategies rather than true comprehension of visual rules. The overall performance of VLMs remained significantly lower than that of humans, particularly in tasks requiring spatial relations and concept generalization.
Applications
This research has significant implications for developing more capable VLMs and their potential in various fields. Understanding current VLM limitations provides insights that can guide future AI innovations, especially in enhancing multimodal reasoning capabilities. Improved VLMs could be applied in areas such as advanced autonomous systems, robotics, and interactive technologies in human-computer interaction, where interpreting and reasoning about visual data is crucial.
The authors also highlighted the need for more research in multimodal AI, particularly in refining models to better understand and generalize complex visual concepts. Future innovations that address the identified limitations could work toward creating AI systems that closely mimic human cognitive abilities, expanding their applicability across diverse domains.
Conclusion and Future Directions
In summary, the study provided valuable insights into the current state of VLMs and their performance on Bongard Problems. The study underscores a significant gap between human-like reasoning and machine cognition, especially in visual understanding.
As VLMs evolve, addressing the identified limitations will be essential for enhancing their capabilities and expanding their applications in practical, real-world scenarios. Future work should focus on developing advanced models that can better comprehend and reason about visual concepts, ultimately bridging the gap between human intelligence and machine learning.

*Important notice: arXiv publishes preliminary scientific reports that are not peer-reviewed and, therefore, should not be regarded as definitive, used to guide development decisions, or treated as established information in the field of artificial intelligence research.
Journal reference:
- Preliminary scientific report.
Wüst, A., Tobiasch, T., Helff, L., Dhami, D. S., Rothkopf, C. A., & Kersting, K. (2024). Bongard in Wonderland: Visual Puzzles that Still Make AI Go Mad? ArXiv. https://arxiv.org/abs/2410.19546