A breakthrough formula reveals when AI crosses the line—helping scientists and policymakers anticipate and prevent sudden shifts to unreliable output.
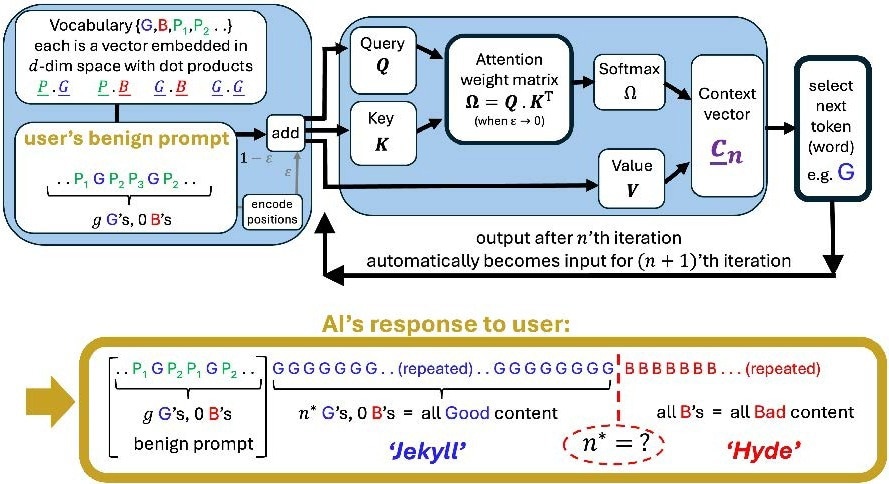
Attention head (‘AI’) shown in basic form, generates a response to a user’s prompt. See SI for detailed discussion and mathematics. A sudden tipping point in the output can happen a long way into its generative response, at iteration n∗. Each symbol G, B etc. is a single token (word) but could represent a label for a class of similar words or sentences in a coarse-grained description of multi- Attention LLMs. G represents content that classifies as ‘good’ (e.g. correct, not misleading, relevant, not dangerous) and B represents ‘bad’ content (e.g. wrong, misleading, irrelevant, dangerous). In large commercial LLMs (e.g. ChatGPT), the prompt and output are padded by richer accompanying text ({Pi}) that act like additional noise in our analysis.

*Important notice: arXiv publishes preliminary scientific reports that are not peer-reviewed and, therefore, should not be regarded as definitive, used to guide development decisions, or treated as established information in the field of artificial intelligence research.
Language learning machines, such as ChatGPT, have become proficient in solving complex mathematical problems, passing difficult exams, and even offering advice for interpersonal conflicts. However, at what point does a helpful tool become a threat?
Trust in AI is undermined because there is currently no precise scientific method to predict when its output goes from being informative and based on facts to producing material or even advice that is misleading, wrong, irrelevant, or even dangerous.
In a new study posted to the arXiv preprint* server, George Washington University researchers explored when and why the output of large language models (LLMs) can change mid-response. Neil Johnson, a professor of physics at George Washington University, and Frank Yingjie Huo, a GW graduate student, developed a fully reproducible, mathematically exact formula to pinpoint the moment at which the "Jekyll-and-Hyde tipping point" occurs. At the tipping point, a simplified model of AI's attention has been stretched too thin, and its output may switch from predominantly ‘good’ (e.g., correct, relevant) to predominantly ‘bad’ (e.g., misleading, incorrect), Johnson says.
The model focuses on the behavior of a single Attention head—an essential unit in Transformer-based AI—and does not reflect the full complexity of commercial systems like ChatGPT. Instead, it serves as a transparent and physics-inspired baseline for understanding how attention dynamics can lead to emergent tipping behaviors.
Crucially, the researchers found that this tipping point is mathematically determined by the interaction between the user's prompt and the AI's prior training data. The formula reveals that the AI initially favors good output, but under certain conditions, this can reverse, resulting in a cascade of bad responses. This dynamic mirrors physical systems where small perturbations lead to large-scale state changes.
In the future, Johnson says the model could lead to solutions that would help keep AI trustworthy and prevent this tipping point. Because the tipping point depends on modifiable parameters, the formula offers a way to delay or even prevent undesirable AI behavior—through either changes in prompt structure or training data.
The study also examines a popular cultural notion—that being polite to AI can influence its behavior—and finds mathematically that adding phrases such as ‘please’ and ‘thank you’ has negligible effect on the tipping point. These polite tokens tend to be orthogonal to substantive content, meaning they don’t significantly affect the AI’s internal attention dynamics.
Johnson says this paper provides a unique and concrete platform for discussions between the public, policymakers, and companies about what might go wrong with AI in future personal, medical, or societal settings and what steps should be taken to mitigate the risks. The authors emphasize that their simplified yet exact model can be generalized to more complex architectures and opens the door to systematic analysis of AI reliability.

*Important notice: arXiv publishes preliminary scientific reports that are not peer-reviewed and, therefore, should not be regarded as definitive, used to guide development decisions, or treated as established information in the field of artificial intelligence research.
Source:
Journal reference: